The need for high-quality, regularly updated and cost-efficient digital data is rising rapidly. We are not far from the everyday reality of digital twins, autonomous vehicles and fully holistic smart cities.
Infrastructure digitization has been an ongoing process for a long time, but now it is happening exponentially. Pivoting from manual labor to automated solutions makes digitization efficient and viable in many applications. Digital data is applicable in numerous use cases:
- Smart Parking – Point cloud maps are used to automate parking.
- Traffic Management – Regularly updated and classified GIS maps allow for better efficiency and planning.
- Project and Operations Management – Accurate data via modern MMS solutions makes infrastructure projects and project management cheaper and quicker.
- Road Safety, – Maintenance and – Asset Management – Digitized environments enable road authorities to track road degradation, calculate costs, analyze vast amounts of road infrastructure data fast with AI classification and AI defect detection. Keeping track of assets and reacting to problematic situations is a must for local and municipal entities.
- Prediction and Prevention Management – EyeVi allows, through our analytical pipeline, to predict future costs, maintenance and prevent traffic situations before they can escalate to reality.
How?
At EyeVi, our mission is to make 3D mapping of road networks accessible and infrastructure management sustainable. Infrastructure digitalisation is in progress worldwide, and this is how we do it.


Point clouds are classified with custom AI algorithms (and manually checked/corrected). The classified objects include road surfaces, ground, buildings, posts, road signs, guard rails, barriers, etc.
To process and digitize raw data collected by the Mobile Mapping System (orthophotos, panoramic images, point clouds), we employ AI-based image and point cloud recognition techniques.These tasks executed by AI include but are not restricted to:
- Detection of drivable road area and road attributes from orthophotos
- Identification of pavement defects in orthophotos
- Detection of traffic signs in panoramic images
- Classification of objects (posts, buildings, vegetation, etc.) in point clouds
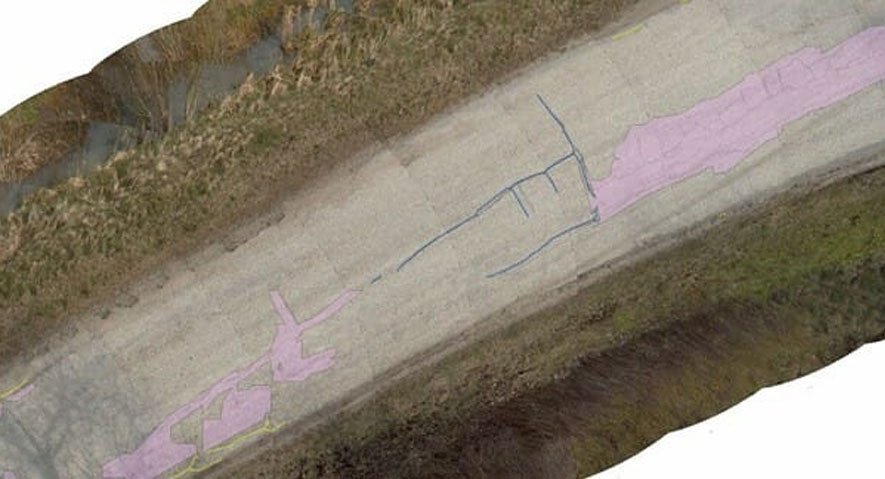

Presently, AI aims to perform tasks that are characteristic of human intelligence. This involves tasks like planning, understanding language, recognizing objects and sounds, learning, and problem-solving. Such AI exhibits some facet of human intelligence and can do that facet well, but lacks other areas. A way of achieving AI is machine learning, which is characterized by the ability to learn without being explicitly programmed. Deep learning of convolutional multilayer neural networks (CNNs) that employ 2D or 3D filters is one of many machine learning approaches. The drawback of this approach is that we need a large quantity of annotated source data to teach the network. When trained, CNNs can perform classification, semantic segmentation, object detection, or instance segmentation of 2D or 3D data.